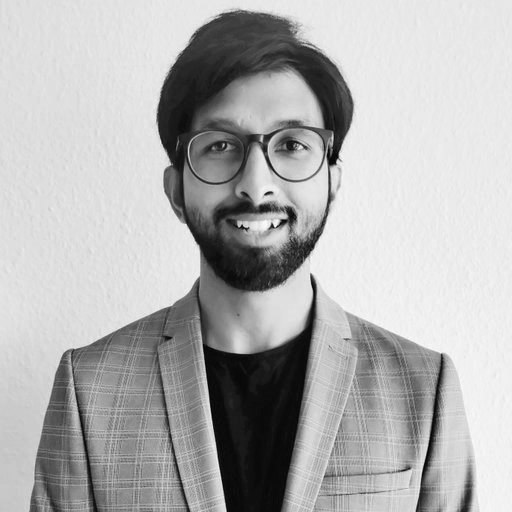 |
Ujwal Gadiraju is a tenured Assistant professor in the Software Technology Department of the faculty of Electrical Engineering, Mathematics, and Computer Science at Delft University of Technology in the Netherlands. He co-directs the TU Delft “Design@Scale” AI Lab and is a member of the program management team of the TU Delft AI Labs. He is the Vice-Chair of CHI Netherlands, representing the human-computer interaction community of academics and industry practitioners in the Netherlands, and has served as an ACM Distinguished Speaker. Ujwal leads a research line on Human-Centered AI and Crowd Computing, actively collaborating with experts in healthcare, finance, and education and working with a variety of industry partners and NGOs to tackle important societal problems. Before joining the WIS group, Ujwal worked at the L3S Research Center as a Postdoctoral researcher between 2017-2020. He received a PhD degree (Dr. rer. nat.) in Computer Science with a summa cum laude recognition from the Leibniz University of Hannover, Germany, in 2017, an MSc. Computer Science degree from TU Delft, the Netherlands, in 2012, and a B.Tech. Computer Science and Engineering degree from VIT University, India in 2010. His research interests lie at the intersection of Human-Computer Interaction (HCI), Artificial Intelligence (AI), and Information Retrieval (IR). Ujwal has published over 200 peer-reviewed articles in these fields. His work has been recognized with several honors, including 10 paper awards at top-tier HCI and AI conferences. His current research focuses on creating novel methods, interfaces, systems, and tools to overcome existing challenges on the path toward building more effective and inclusive AI systems and facilitating appropriate reliance of humans on such systems.
|
|
More about Ujwal Gadiraju topics
Ujwal will present two distinct sessions during the Winter School: Session B1 and B2.
Session B1 - Fostering Appropriate Trust and Reliance in Human-AI Decision-making
Advances in AI and machine learning technologies have snowballed the proliferation and adoption of AI systems across different domains ranging from finance to health and education. Researchers and practitioners in different communities exploring the societal impact of integrating AI systems in our everyday lives have recognized the dangers of over-trust and blind reliance on AI systems. In equal measure, there has been a recognition of the potential benefits in collaborating with AI systems that can aid humans in domains and contexts that go beyond their expertise or otherwise complement human capabilities. Striving to foster appropriate reliance (i.e., simultaneously preventing over-reliance or under-reliance) on AI systems has been akin to walking a tight rope. Over the last decade, several methods and interventions have been proposed to this end, but with limited success. This lecture will present an overview of the empirical pursuit of facilitating appropriate reliance in human-AI decision-making and the lessons we learned along the way. The lecture will also discuss the open opportunities and challenges that lie ahead of us in the imminent future.
Session B2 - Designing Effective Conversational Interfaces for Human-AI Collaboration
The rise in popularity of conversational agents has enabled humans to interact with machines more naturally. There is a growing familiarity among people with conversational interactions mediated by technology due to the widespread use of LLM agents, mobile devices, and messaging services. Over half the population on our planet has access to the Internet with ever-lowering barriers to accessibility. Though text modality is a dominant way to implement conversational user interfaces (CUIs) today, foundational AI models enable the implementation of multimodal CUIs using voice and visual modality. Adopting visual and auditory cues in addition to text-based responses provides an engaging user experience, specifically in complex scenarios like health guidance, and job interviewing, among others. This lecture will present a review of state-of-the-art research and best practices on building and deploying multimodal CUIs and synthesize the open research challenges in supporting such CUIs. The lecture will also showcase the benefits of employing novel conversational interfaces in the domains of human-AI decision-making, health and well-being, information retrieval, and crowd computing. The lecture will also discuss the potential of conversational interfaces in facilitating and mediating the interactions of people with AI systems.
|